일 | 월 | 화 | 수 | 목 | 금 | 토 |
---|---|---|---|---|---|---|
1 | 2 | 3 | ||||
4 | 5 | 6 | 7 | 8 | 9 | 10 |
11 | 12 | 13 | 14 | 15 | 16 | 17 |
18 | 19 | 20 | 21 | 22 | 23 | 24 |
25 | 26 | 27 | 28 | 29 | 30 | 31 |
- 아이폰원스토어
- 선대
- ㅐㅕ세ㅕㅅ
- adversarialattackonmonoculardepthestimation
- 백준
- MacOS
- 맥북원스토어
- BOJ
- CS231nAssignment1
- Linear algebra
- pycharmerror
- ios원스토어
- CNN구조정리
- BAEKJOON
- CS231nSVM
- CS231n
- 백준알고리즘
- professor strang
- Algorithm
- monoculardepthestimation
- CS231ntwolayerneuralnet
- CNNarchitecture
- arm칩에안드로이드
- 선형대수학
- 맥실리콘
- CS231nAssignments
- MIT
- Gilbert Strang
- RegionProposalNetworks
- gpumemory
- Today
- Total
목록Study (22)
개발로 하는 개발
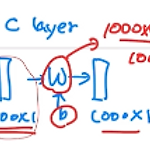
CNN( = ConvNet) - sequence of layers - each layer of a ConvNet transforms one volue of activations to another through a differientable function - one volume of activations = activation map = feature map ReLU(nonlinear) layer : activates relevant responses Fully-Connected Layer : each neuron in a layer will be connected to all the numbers in the previous volume Pooling Layer : downsampling operat..
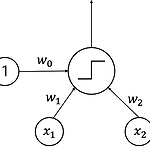
1. Deep Neural Network (심층신경망) - Perceptron : Linear classifier $y = f(w_{0} + w_{1}x_{1} + w_{2}x_{2})$ non-linear한 function을 계산하기 위해서는? multi-layer perceptron을 이용한다. - hidden layer : N-Layer Neural Network = (N-1)-hidden-layer Neural Network - Forward Propagation : Wx +b 2. Training Neural Networks - Gradient Descent : 일반적으로는 Adam이라는 방법이 최적 : local minima의 문제가 생길 수 있음 : ground truth 값에 대한 loss..
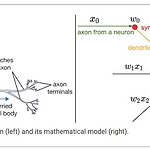
Two Layer Net biological neuron vs mathematical input&output of neuron - activation function (or non-linearity) takes a single number and performs a certain fixed mathematical operation on it - fully-connected layer in which neurons between two adjacent layers are fully pairwise connected, but neurons within a single layer share no connections - Each Layer usually matrix multiplication with acti..
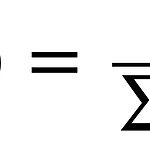
Softmax Another popular classifier (like SVM) Generalized version of binary Logistic Regression classifier - Softmax function : - Loss function : cross-entropy function - Numerical Stability : exponential -> very large number -> normalize values - SVM vs Softmax Same score function Wx = b, different loss function. Softmax : Calculate probabilities for each classes. Easier to interpret. Softmax.p..
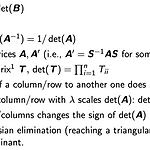
1. Determinant - Definition For $A=\begin{pmatrix}a_{11}a_{12}\\a_{21}a_{22}\end{pmatrix},A^{-1}=\frac{1}{a_{11}a_{12}-a_{21}a_{22}}\cdot\begin{pmatrix}a_{22}-a_{12}\\-a_{21}a_{22}\end{pmatrix}$ A is invertible if $\frac{1}{a_{11}a_{22}-a_{21}a_{12}}\neq 0$ $\therefore det(A) = \begin{vmatrix}a_{11}a_{12}\\a_{21}a_{22}\\\end{vmatrix} = \frac{1}{a_{11}a_{12}-a_{21}a_{22}}$ - for $3\times3$ matrix..
1. 데이터의 분석 - 인과관계에 따른 데이터 분석 (상관관계 x) - 데이터 전처리 : outlier 제거, 데이터 표준화 - 데이터 분석 : Error bar, 충분한 EDA(exploratory data analysis) - 데이터의 양 : Under-fitting / Over-fitting - training data != test data 2. Black box algorithm - deep learning 의 특성 : 왜 이런 결과가 나왔는지 설명하지 못하는 경우, 실제로 이를 적용하기 힘들 수 있음 - 성능 vs 설명력 - post-hoc explanability - one-pixel attack : 한 픽셀 차이로 결과가 변화할 수 있는 가능성. noise 문제 해결의 중요성 3. Web ..
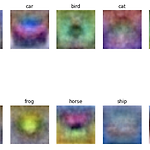
KNN - space inefficient : have to remember all the data in the training set - classifying is expensive : must calculate all the distances to all of the training set -> Use SVM SVM Linear Classification - Score function, Loss function 사용 : minimize the loss function with respect to the parameters of the score function. CIFAR-10 we have a training set of N = 50,000 images, each with D = 32 x 32 x ..
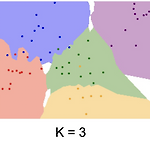
- KNN ( K nearest neighbor) hyperparameter : k, L1 or L2 (distance calculating formula) Basically, you are trying to figure out which dot belongs to what region. And you are determining this by calculating the distance between the test point and train points. You get the value of k nearest points, and decide whichever the majority is. There are two ways to calculate this. L1 and L2. in the assig..